SynSurr: Empowering Genome-Wide Association Studies by Overcoming the Challenges of Missing Phenotype Data
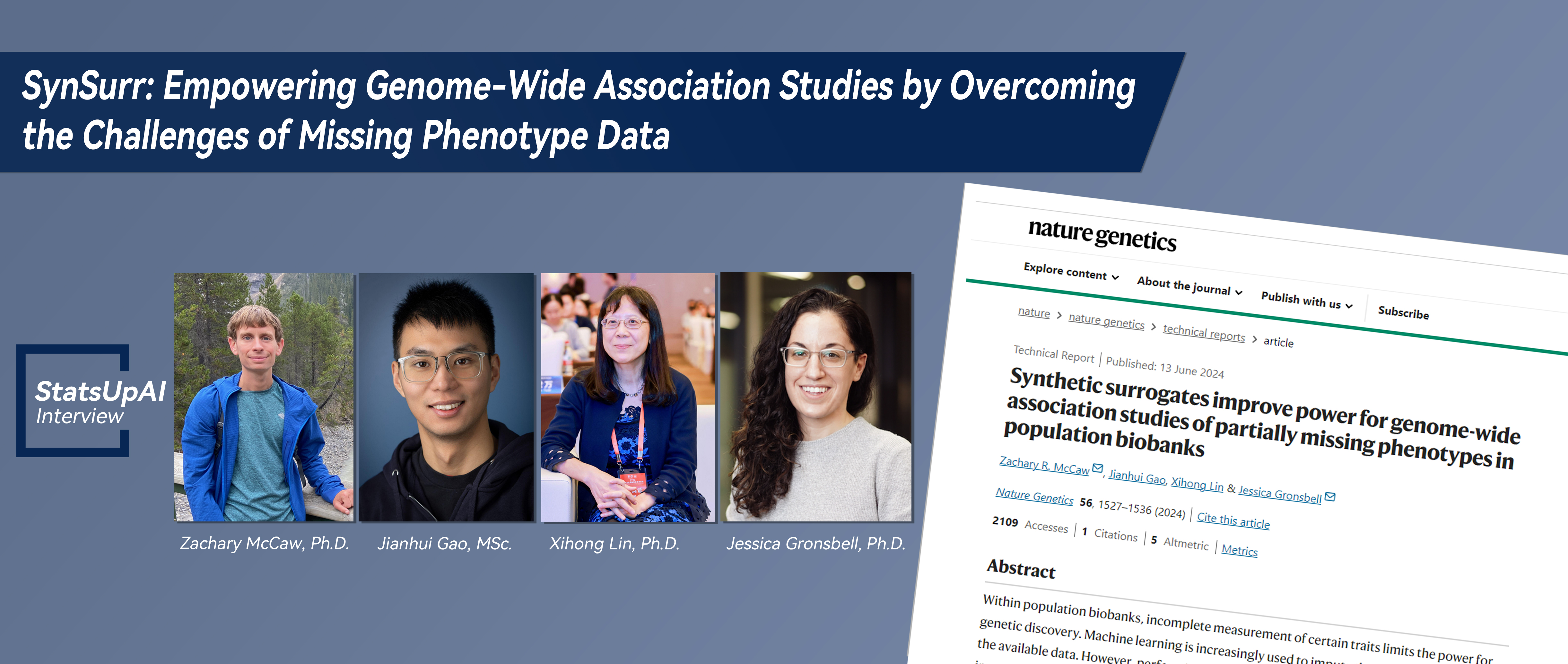
The Article Link:
Synthetic surrogates improve power for genome-wide association studies of partially missing phenotypes in population biobanks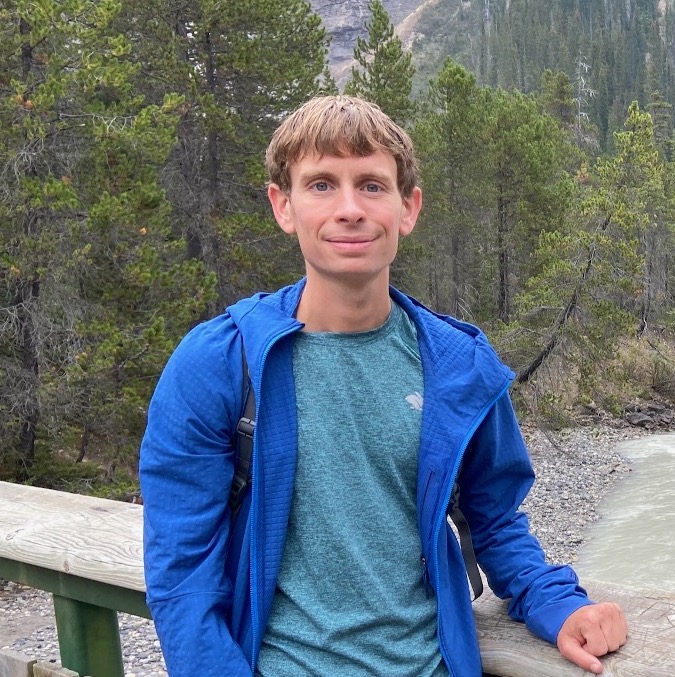
Dr. Zachary McCaw
Dr. McCaw is a Staff Machine Learning Scientist at Insitro. His research focuses on combining statistics with machine learning to improve genetic discovery and precision medicine. Previously, he completed his Ph.D. in Biostatistics with Professor Xihong Lin at Harvard.
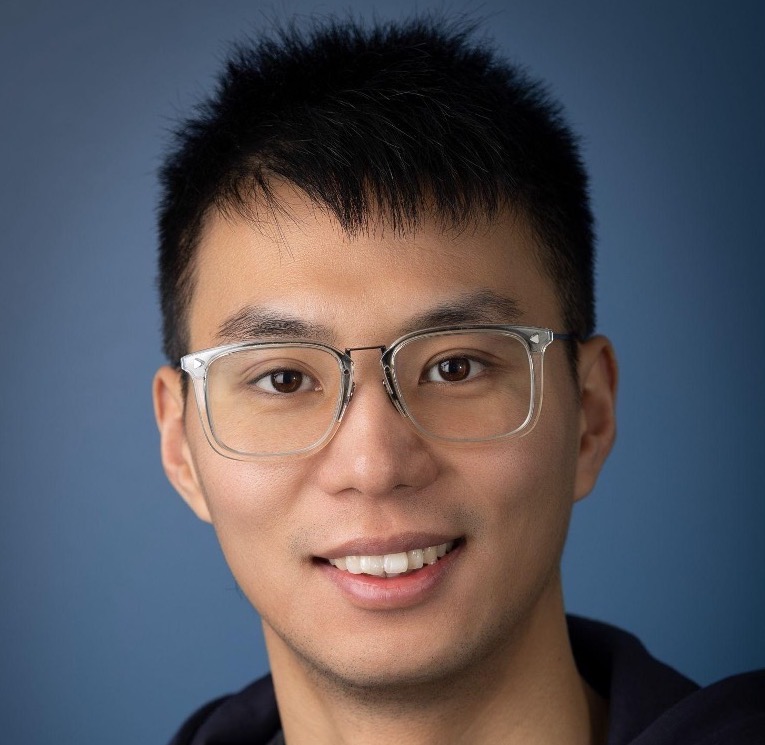
Jianhui Gao, MSc.
Mr. Gao is currently a PhD candidate in the Department of Statistical Sciences at the University of Toronto, under the supervision of Professors Jessica Gronsbell and Lei Sun. His thesis focuses on developing semi-supervised methods for analyzing various sources of health data, including electronic health records and genetic data.
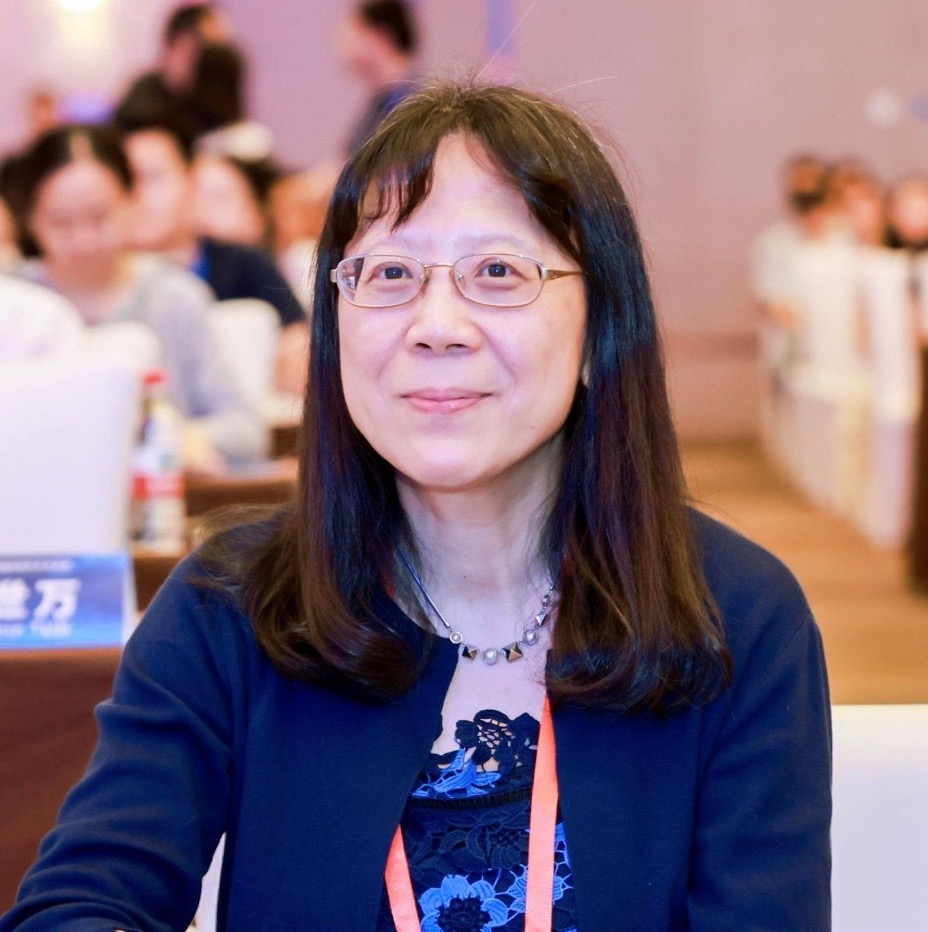
Dr. Xihong Lin
Dr. Lin is Professor and former Chair of Biostatistics, and Coordinating Director of the Program in Quantitative Genomics at Harvard School of Public Health, and Professor of Statistics at Harvard University. Dr. Lin works on the development and application of scalable and interpretable statistical and machine learning methods for analysis of big and complex genomic and health data, including multi-ethnic biobanks, whole genome sequencing studies, and multi-omics data. Dr. Lin is an elected member of the US National Academy of Medicine and the US National Academy of Sciences. She received the 2002 Mortimer Spiegelman Award from the American Public Health Association, the 2006 Presidents’ Award from the Committee of Presidents of Statistical Societies (COPSS). She also received the 2017 COPSS FN David Award, the 2022 National Institute of Statistical Sciences Sacks Award for Outstanding Cross-Disciplinary Research, and the 2022 Zelen Leadership in Statistical Science Award. She is an elected fellow of American Statistical Association, Institute of Mathematical Statistics, and International Statistical Institute. Dr. Lin’s research has been supported by the MERIT Award (2007-2015) and the Outstanding Investigator Award (2015-2029) from the National Cancer Institute. Dr. Lin is the former Chair of COPSS and is the former Editor of several biostatistical journals.
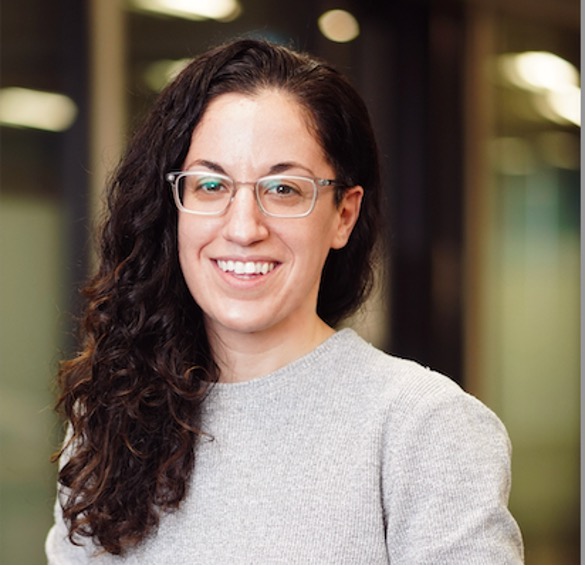
Dr. Jessica Gronsbell
Dr. Gronsbell is an Assistant Professor in the Department of Statistical Sciences at the University of Toronto, with cross-appointments in the Departments of Family and Community Medicine and Computer Science. Her research focuses on developing statistical learning and inference methods to tackle key challenges in analyzing modern observational health data, particularly electronic health records data.
Regarding the research background and significance, does this work discover new knowledge or solve existing problems within the field? Please elaborate in detail.
Synthetic surrogate analysis, or SynSurr, was developed to address the problem of missing data in genome-wide association studies (GWAS). Within large population biobanks, such as the UK Biobank (~500,000 participants), certain phenotypes of interest, especially those that require specialized imaging, are only measured for a subset of the cohort. Examples include body composition as measured by dual-energy X-ray absorptiometry, neurological and cardiac structure as measured by magnetic resonance imaging, and optical parameters as measured by color fundus photography, among others. In recent years, the practice of developing machine learning (ML) models to impute the missing values of these specialized phenotypes has become commonplace. However, performing GWAS on an imputed outcome carries an increased risk of discovering false-positive associations. SynSurr proposes a new approach for leveraging an ML-derived surrogate phenotype, which we describe as a “synthetic surrogate”, to empower GWAS of a partially missing target phenotype. Rather than replacing missing values with imputations from an ML model, or analyzing the ML-phenotype in place of the target phenotype, SynSurr jointly analyzes the target phenotype and its synthetic surrogate within a bivariate outcome framework. We show that SynSurr is unbiased, estimating the same effect size as standard GWAS of the target phenotype, properly controls the type I error, and improves power in proportion to squared correlation between the target and ML phenotypes. Importantly, unlike existing methods for imputation-based inference, the validity of SynSurr does not depend on proper specification of the imputation model. In fact, SynSurr remains unbiased and comparably powerful to standard GWAS even when the ML-phenotype is unrelated to the target phenotype.
How did the reviewers evaluate (praise) it?
Reviewers thought that the problem addressed by SynSurr was an important and practical one often encountered when analyzing large scale biobanks, such as the UK biobank, that the method was simple but innovative, that the pitfalls of existing approaches provided compelling motivation for SynSurr’s development, that the statistical validation was rigorous, that the power advantage achieved in the real-data application was impressive, and overall that the paper was clearly written and explained. Nature Genetics publishes reviews alongside the paper, which can be found with the online manuscript.
If this achievement has potential applications, what are some specific applications it might have in a few years?
The most immediate application of SynSurr is to provide a valid method of improving power for GWAS of phenotypes that are measured in only a subset of any large population biobank. We demonstrate an application of SynSurr to GWAS of dual X-ray absorptiometry (DEXA) measurements of body-composition in the UK biobank data, borrowing power from an ML-derived surrogate phenotype based in part on bioelectrical impedance. More generally, the SynSurr framework provides a simple alternative to imputation that enables valid inference on a partially missing target outcome without requiring that the imputations be generated from a correctly specified model.
Can you recount the specific steps or stages from setting the research topic to the successful completion of the research?
SynSurr was inspired by the observation that, in the context of large biobanks, such as the UK Biobank, ML-models are increasingly capable of predicting missing phenotypes, yet guidance was lacking on how to perform GWAS on those model’s predictions. Proxy GWAS, in which the predicted phenotype is analyzed in place of the target phenotype, is sometimes reasonable, but becomes questionable when the quality of the predictions is only modest. Multiple imputation, in which missing values of the target phenotype are repeatedly generated from a probabilistic model, provides an alternative, but is sensitive to correct specification of the imputation model. Prior to SynSurr, no method was widely available for leveraging a predicted phenotype to empower GWAS of a partially missing target phenotype while also guaranteeing 1) estimation of the same effect size as standard GWAS, 2) proper control of the type I error, and 3) robustness to specification of the imputation model. We set out to fill this gap. Our first steps were to propose the model then derive and implement the estimation and inference procedures. We next conducted extensive simulation studies to validate SynSurr’s operating characteristics. To build further conviction, we designed an ablation study, using data from the UK Biobank, where analyses were performed while masking a progressively larger proportion of the target phenotype. This enabled us to compare the performance of SynSurr, and its comparators, to an oracle model that had access to the complete data. Finally, having validated SynSurr on both simulated and real data, we applied our method to empower GWAS of several body composition phenotypes, which were measured via DEXA scan in only a subset of the UK Biobank.
Were there any memorable events during the research? You can tell a story about anything related to people, events, or objects.
A pivotal moment in the development of SynSurr was seeing the results from its application to the DEXA body composition phenotypes in the UK Biobank. From our previous simulation and ablation studies, we knew SynSurr worked in principle, yet the magnitude of the benefit it would provide in practice remained uncertain. Fortunately, SynSurr exceeded our expectations, providing a nearly 22-fold improvement in the number of genome-wide significant associations, from a mean of 8 with standard GWAS to 180 with SynSurr. Investigation of SynSurr’s lead variants confirmed that many had existing support from the literature, and that the tagged genes were highly enriched for pathways related to body composition and anthropomorphic traits.
Is there a follow-up plan based on this research? If so, please elaborate.
We are working on an extension of SynSurr to the rare-variant setting. Our initial method focuses on identifying associations between individual common variants and a target phenotype. Different methodology is required for rare variants, which may be present in only one or two subjects even in biobank-scale cohorts. The extension will focus on leveraging an ML-phenotype to improve power for identifying an association between the collection of rare variants in a given gene and functional category, and the target phenotype.
Without a doubt, AI is one of the hot topics of 2024, requiring extensive data support in its development. What assistance can biostatistics offer to the development of AI?
SynSurr provides a quintessential example of how biostatistics and AI or ML can intersect. While AI/ML provides an unparalleled ability to generate predictions, questions of statistical inference and estimation remain paramount. For example, whether and to what extent a model’s outputs are related to other variables (such as genotype) is scientifically important. SynSurr further distinguishes association with the target outcome (i.e., what the ML model is trying to predict) from association with the model’s predictions. While the two are related, they are not identical, especially when the model’s predictions are imperfect. Statistical thinking has an important role to play in highlighting distinctions such as these, and asking how the outputs of AI/ML models can be leveraged to benefit well-known estimation and inference tasks, as we did in SynSurr.
Proofread by: Hongtu Zhu