Interview with Award Winner Dr. Yize Zhao for the Interface of Statistics and Al
Interviews
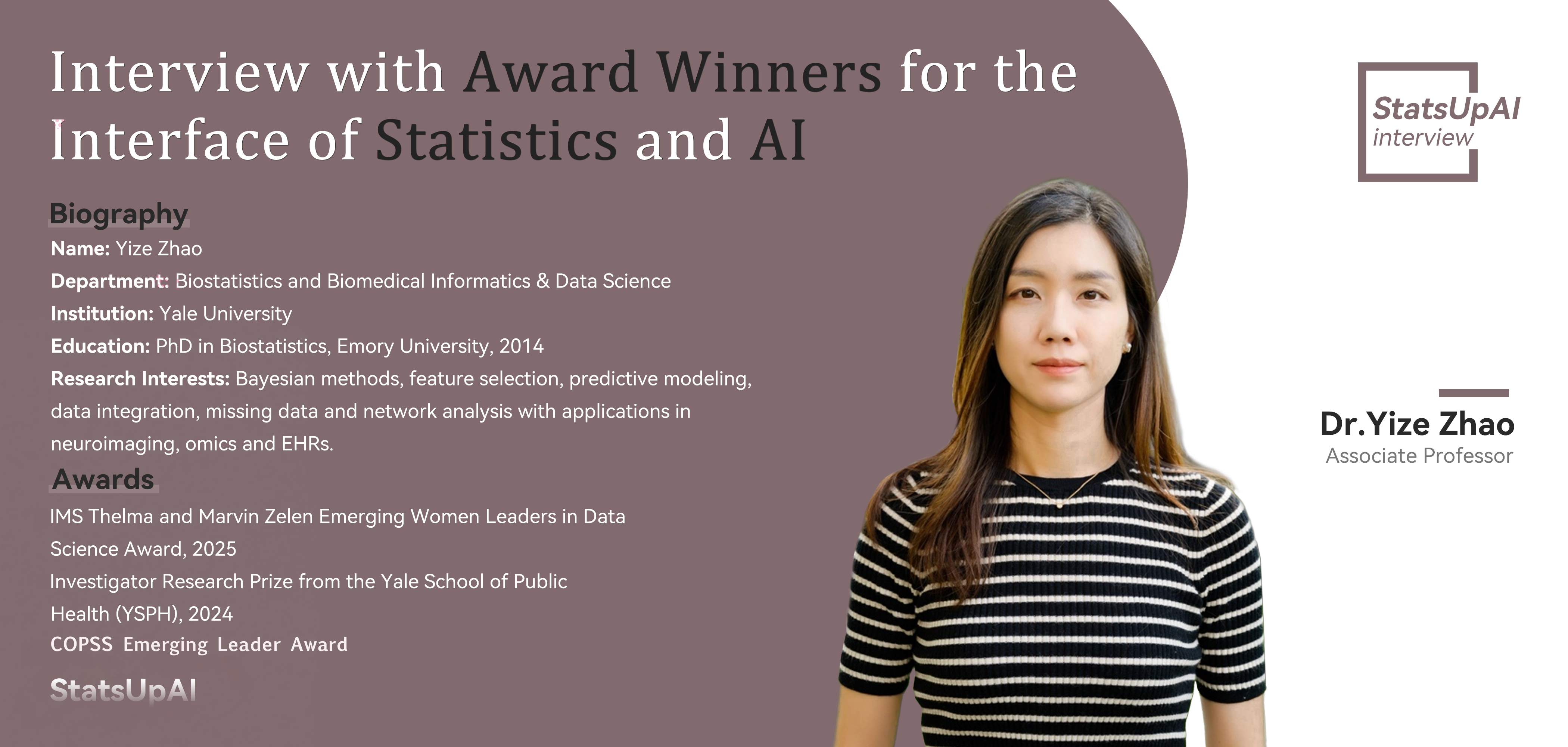
1. Can you summarize your award-winning research and its significance for statistics and AI?
I am honored to receive the IMS Thelma and Marvin Zelen Emerging Women Leaders in Data Science Award for my research on innovative and interpretable Bayesian modeling and computation for structural and graphical data. My work focuses on developing advanced analytical methods and applications in medical imaging, particularly integrating imaging data with other biomedical and real-world data sources to gain meaningful insights.
Brain imaging data presents unique challenges due to its high noise levels, complex topological structure, multi-modal nature, and intricate relationships with other data sources, such as molecular and behavioral data. These complexities make it challenging to extract meaningful signals from imaging scans and to better understand psychiatric disorders and neurodevelopmental or aging processes. To address these challenges, my group has developed a series of supervised and unsupervised learning models that account for heterogeneity across data sources and accommodate diverse variable types, including network-based brain connectivity measures. Our models are designed to reflect underlying neurobiological mechanisms by incorporating biologically-informed modeling for features such as hub nodes and modular structures. This ensures that our findings are not only statistically rigorous but also biologically interpretable, offering valuable insights into the relationships between genetic factors, brain signatures, and behavioral outcomes.
The significance of my work for both statistics and AI lies in bridging the gap between neuroscience and advanced analytical methods. By grounding model complexity in biologically driven principles, we enhance both the interpretability and practical applicability of AI-driven approaches in neuroscience and mental health research. This interdisciplinary framework paves the way for more accurate and reliable insights that can inform clinical decision-making and advance our understanding of the brain.
2. What statistical methods from your research are most applicable to AI?
One of the key areas my group has been actively working on is Bayesian deep learning, where we focus on incorporating prior knowledge and principled uncertainty quantification into deep learning models. To address the inherent black-box nature of conventional deep learning models, my research develops analytical frameworks grounded in Bayesian inference. Our work integrates CNN and transformer models to generate posterior distributions for complex latent structures that are often difficult to accurately estimate using traditional posterior computation methods. These Bayesian frameworks enable rigorous inference for key learning parameters, enhancing the interpretability and reliability of decision-making processes. I am particularly passionate about bridging the gap between traditional statistical learning approaches and modern AI models, striving to achieve a balance that leverages the strengths of both paradigms.
Another significant area of my research involves higher-order relationship modeling, which aims to capture complex interactions within high-dimensional data. Analyzing these relationships presents challenges, particularly due to the exponential search space required to explore all potential interactions. To address this, my group has developed a novel information bottleneck framework that efficiently identifies maximally informative and minimally redundant higher-order relationships. This approach builds on foundational statistical principles to ensure rigorous variable selection and feature representation, ultimately enhancing model interpretability and improving generalizability in high-dimensional settings. Again, this represents a combination of statistical rigor with modern computational techniques to provide scalable and reliable solutions for analyzing complex data structures.
3. How does your work address challenges at the intersection of statistics and AI?
My work addresses key challenges at the intersection of statistics and AI by enhancing the interpretability, reliability, and theoretical foundation of AI models while ensuring they remain computationally efficient and applicable to complex real-world problems. One major challenge in this field is balancing the flexibility of AI models with the rigorous inferential guarantees offered by statistical methods. To address this, my research focuses on embedding core statistical principles—such as uncertainty quantification and structured inference—into AI frameworks. This integration helps ensure that model outputs are not only accurate but also informative and trustworthy. Another critical challenge involves scaling statistical methods to handle high-dimensional and unstructured data. My work focuses on developing computational strategies that enable statistical models to process large datasets efficiently while maintaining their interpretability and inferential integrity.
Additionally, my research emphasizes the importance of integrating domain knowledge into AI models to enhance their practical relevance and ensure meaningful insights. By leveraging AI techniques with modern statistical frameworks, my team is working to develop models that are both theoretically sound and adaptable to evolving data challenges, and ultimately providing more reliable and interpretable outputs.
4. What impact do you foresee your research having on the future of AI systems?
I see my research contributing to the future of AI systems by helping them evolve into more trustworthy, adaptable, and knowledge-driven tools. As AI applications continue to grow across various fields, the demand for models that are not only accurate but also interpretable and robust will become increasingly critical. My work focuses on addressing these needs by developing frameworks that integrate statistical reasoning with AI, enabling models to provide meaningful insights beyond just predictions.
One key impact I foresee is improving AI's ability to make well-informed decisions under uncertainty, particularly in the analysis of complex biomedical data. Brain imaging data along with their integration with molecular and real-world data sources like electronic health records, present significant challenges due to their high-dimensional, noisy, and heterogeneous nature. My research focuses on embedding statistical inference within deep learning frameworks to better handle these complexities, allowing AI systems to provide more reliable and interpretable outputs. This approach enhances the ability to identify meaningful patterns and relationships across multiple data modalities, leading to more informed decision-making on disease early diagnosis, prevention and intervention.
5. What emerging trends in statistics do you believe are crucial for advancing AI?
I personally feel the following emerging trends or existing techniques in statistics are crucial for advancing AI, particularly in making models more interpretable, robust, and applicable to complex real-world problems. The first one is the integration of uncertainty quantification in AI models. As AI is widely adopted across various domains, the ability to quantify and communicate uncertainty is essential for informed decision-making. Statistical approaches including Bayesian statistics and inference provide principled frameworks to incorporate uncertainty, enabling AI models to produce more reliable and trustworthy results. The second is the integration of heterogeneous data sources, which is becoming increasingly relevant as AI tackles complex problems that require to analyze diverse data types such as imaging, genetics, and real-world data. Statistical methods that facilitate multi-source data integration—such as latent variable approaches and graph-based techniques—are critical to ensure that AI models can effectively leverage the richness of these data while maintaining interpretability and consistency. Finally, the advancement of explainable AI through causal inference is becoming increasingly important. As AI models grow more complex, understanding the underlying relationships within the data is crucial for generating actionable insights. Statistical causal inference offers a principled way to uncover causal relationships, offering a foundation for building AI systems that go beyond correlation-based learning to better understand the effect mechanisms driving observed patterns.
Edited by: Shan Gao
Proofread by: Jian Kang, Hongtu Zhu
Proofread by: Jian Kang, Hongtu Zhu
Page Views: