Interview with Award-Winning Dr. Weijie Su on the Interface of Statistics and AI
Interviews
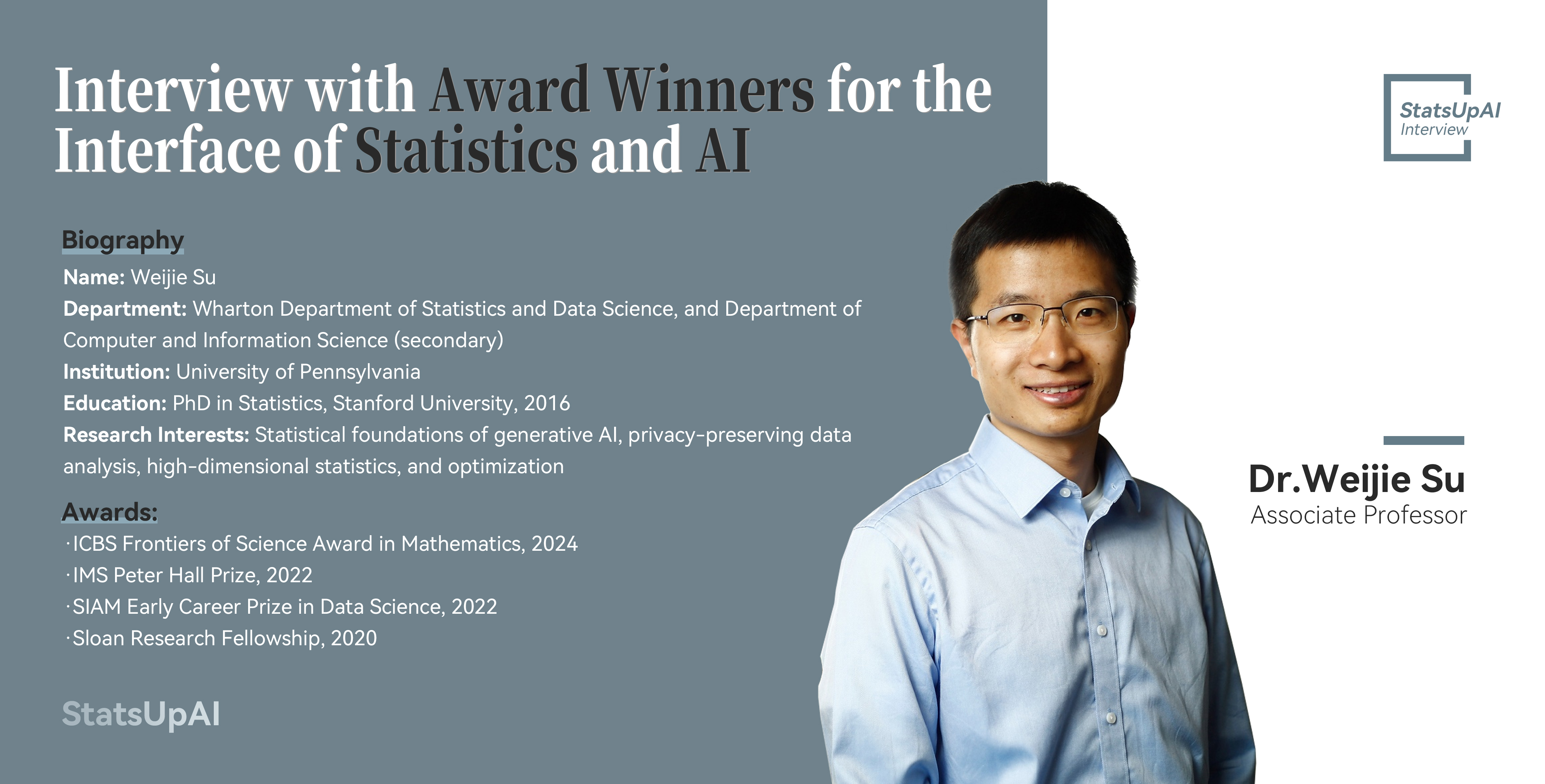
1. How did you first get into AI and start working at the intersection of statistics and AI?
Thank you for the opportunity to share my experience! My PhD training was in high-dimensional statistics and multiple hypothesis testing. However, I started regularly hearing people talk about AI around 2016. At that time, AI mostly referred to multilayer neural networks—very effective, yes, but not exactly what I’d call intelligent. It felt more like a buzzword that companies used, often for marketing purposes.
But everything changed in 2017 with the introduction of Transformer. Suddenly, breakthroughs that had seemed years away were happening—AlphaFold, ChatGPT, and many more. I decided to shift my research focus to AI after playing with ChatGPT in early December 2022. I had this strong instinct that a revolution was beginning. To my delight, my students were even more excited about large language models than I was! Together, we dove into this new direction, approaching it from a statistical perspective.
After nearly two years of working on LLMs, I’m continually amazed at how powerful statistical techniques and insights are in AI research. LLMs and the Transformer architecture are massive and full of complex engineering details. But the beauty of statistics is that we can treat these systems like black boxes and study their probabilistic behavior without needing to untangle the engineering complexities.
2. What statistical methods from your research are most applicable to AI?
My group has been working on LLMs in several directions. One area we’ve been exploring is watermarking for AI-generated content. For example, imagine encountering a piece of text, such as an article or a message, and wondering whether it was written by a human or generated by ChatGPT. Detecting AI-generated text is a critical challenge with wide-ranging applications, from education to combating misinformation. Tech giants like OpenAI and Google DeepMind have been working on watermarking schemes, and DeepMind recently published a Nature paper on their new watermarking method. My group recently developed a statistical framework for watermark detection through the lens of hypothesis testing. By leveraging classical statistical ideas like pivots and robustness, we’ve obtained detection methods that are both efficient and robust.
Another area of our research is addressing fairness challenges in LLM alignment. As LLMs increasingly influence decision-making processes, it is important to ensure that their outputs align with human preferences between, for example, an apple and an orange. However, our recent work reveals that the current training paradigm for LLMs is inherently biased, and we developed a new method that eliminates this bias without adding any computational overhead. Once again, this solution draws heavily on statistical techniques, particularly regularization.
Beyond these applications, my group has also been tackling copyright and privacy challenges associated with generative AI.
While not directly tied to AI, another area of focus for my group has been improving the peer review process for AI/ML conferences. Over the past two years, we’ve experimented at ICML with a new method for estimating review scores for AI/ML conferences. While this isn't directly applicable to AI, it helps create a better academic environment for AI research.
3. What aspects of AI do you think statistics can advance?
Statistics has a tremendous role to play in advancing AI, particularly because generative AI systems like LLMs are inherently probabilistic in nature. Unlike earlier neural networks, which were often deterministic, LLMs generate outputs that are random and probabilistic. This makes them a natural fit for statistical tools and reasoning.
One area where statistics can really help advance is watermarking for LLMs. With the Biden administration’s executive order requiring tech companies to watermark AI-generated content, there’s a real need for robust, provable methods to identify AI-generated outputs. Statistics is great for this because it provides the tools to build efficient and reliable detection systems that can help protect users from fraud and misinformation. Uncertainty quantification is another vital area. LLM outputs are inherently random and often miscalibrated, sometimes being overconfident. This is where statisticians can really make a difference. We're also seeing statistics prove invaluable in LLM alignment, especially regarding distributional properties like fairness, bias, and privacy.
4. What can our community do to get more involved in this AI wave?
This year, I’ve seen so many exciting initiatives in the statistics community that promote AI research. For example, earlier this year, there was a fireside chat on the intersection of statistics and AI. I hope we’ll see more events like this in the future.
Special issues in journals can also inspire statisticians to contribute more to AI. It’s great to see JASA launching a special issue on this topic. I’m also guest-editing a special issue on statistics and LLMs at Stat (please submit your work to us. Thanks!).
We should also modernize statistical education by integrating more AI elements. I had the privilege of teaching a short course on LLMs with colleagues at JSM this year. I’m very encouraged based on the feedback we got, and while it took a lot of time to prepare, it was incredibly rewarding. I plan to offer it again at ENAR and through ASA’s traveling course program.
Looking at the bigger picture, I think we should consider establishing our own AI/ML conference with proceedings. It's not only about the quick turnaround of conference review processes, it's more about accommodating how AI research advances. Many AI advances are enabled first by individual and preliminary findings, which can be challenging to publish in statistics journals, instead of well-established frameworks or comprehensive sets of results. While publishing in ICML and NeurIPS has become very common among young statisticians, these contributions could be easily perceived by the public as CS contributions since these conferences are considered CS venues, even when statisticians produce the results. This has been my own experience as well. Having our own conference with proceedings would help us claim a stronger voice in the AI era.
Edited by: Shan Gao
Proofread by: Hongtu Zhu
Proofread by: Hongtu Zhu
Page Views: